Translate this page into:
Prognostic Potential of MicroRNAs in Glioma Patients: A Meta-Analysis
Address for correspondence Syed Ather Enam, MD, PhD, Department of Surgery, Aga Khan University, Stadium Road, Karachi 74800, Pakistan. ather.enam@aku.edu
This article was originally published by Thieme Medical and Scientific Publishers Pvt. Ltd. and was migrated to Scientific Scholar after the change of Publisher.
Abstract
Introduction MicroRNAs are a noncoding RNA involved in affecting several transcription and translation pathways. Their use has been discussed as potential predictors of several tumors. Their use as potential biomarker in glioma patients is still controversial. The purpose of this meta-analysis is to explore the possible role of such microRNAs in glioma patients.
Methods After an extensive literature search done on PubMed and Embase, 20 studies were chosen for our analyses with the 9 discussing 11 tumor promoting microRNAs and 11 studies discussing 11 tumor suppressing microRNAs. The data needed was extracted from these studies including the hazard ratio that was used as the effect size for the purpose of our analysis. The needed analysis was performed using Stata and Excel.
Results The pooled hazard ratio for our analysis with patients having a lower microRNA expression for tumor promoting microRNAs came to be 2.63 (p < 0.001), while the hazard ratio for patients with higher expression of tumor promoting microRNA was 2.47 (p < 0.001) with both results being statistically significant. However, as significant heterogeneity was observed a random effect model for analysis was used. Subgroup analysis was further performed using grade, cutoff value (mean or median), sample type (Serum or Blood), and Karnofsky performance score, all of them showing a high hazard ratio.
Conclusion Our results showed that both tumor inhibitory and promoting microRNA can be used as prognostic tool in glioma patients with a poorer prognosis associated with a lower expression in tumor suppressive and higher expression in tumor promoting microRNA, respectively. However, to support this, future studies on a much larger scale would be needed.
Keywords
glioma
microRNA
Introduction
Gliomas comprise a diverse group of central nervous system (CNS) tumors characterized by a pervasive heterogeneity, particularly in terms of their molecular and histological features, as well as their clinical course.1 Regarded as the most frequent primary neoplasm of the brain and spinal cord, gliomas account for 31% of all CNS tumors, and 81% of malignant CNS tumors within the United States, with men having a greater predisposition to developing these lesions.2,3 Traditionally, these tumors have been subdivided according to their semblance to normal glial cells into either astrocytoma, oligodendroglioma, or ependymoma. Since 2007, these lesions were further classified histologically according to morphological features identified on microscopy such as necrosis, mitotic activity, and microvascular proliferation, into grades I through IV as per the 2007 World Health Organization guidelines.4,5 Grades I and II are collectively referred to as low-grade gliomas, while grades III and IV are termed as high-grade gliomas.1,5
Recent advances in molecular analytics have facilitated substantial investigation and discovery of genetic, epigenetic, and molecular profiles of these lesions, precipitating the shift from morphological to molecular diagnosis. This paradigm shift was marked in 2016 when the WHO published revised tumor classification guidelines incorporating these genomic characteristics within the diagnostic criteria.
These advancements in molecular profiling have also yielded a more detailed understanding of various oncogenic and tumor-suppressor proteins. Of particular interest among these are the microRNAs and the role they play in tumor progression and suppression as well as their potential use as prognostic indicators and biomarkers.
MicroRNAs are a class of small noncoding RNAs with most being transcribed from DNA sequences. They play an important role in regulating gene expressions by the pathway affecting the 3′ untranslated region of their target mRNA causing its degradation and translation repression. Although not involved directly in protein coding, they can play a role in tumor pathogenesis.6 Several studies have been conducted to evaluate various microRNA as a prognostic tool, with several these being overexpressed and underexpressed in tumors implying that different microRNA might either promote or suppress tumor suppression, and although still controversial, the possibility of alteration in microRNA expression for cancer treatment has also been discussed.7,8 A meta-analysis done by Li et al looked at prognostic role of microRNA-21 and its role in glioma promotion.33 Several recent studies conducted also highlight microRNAs that play a suppressive role in tumor progression and are downregulated in gliomas especially in those with poorer prognosis; these studies also look at potential role of such microRNA as potential biomarkers for tumor prognosis as well as an option for targeted therapy. Despite all these studies, the prognostic as well as therapeutic role of microRNA with both tumor suppressive and promoter role still remains controversial and studies on it still show inconclusive results. The aim of our study was to do a meta-analysis of such microRNAs to assess their prognostic and therapeutic value. To our knowledge, an analysis of similar nature has not been conducted before.
Materials and Methods
Search Strategy
The guidelines from meta-analysis for observational studies in epidemiology group (MOOSE) were used to carry out the meta-analysis.9 For the purpose of this study, literature search was done on PubMed and Embase from January, 2000 till December, 2020. Both Mesh command and simple Boolean terms were used for the literature search; the Mesh term was (“MicroRNAs”[Mesh]) AND (“Glioma”[Mesh] OR “GBM”[Mesh] OR “Glioblastoma”[Mesh])) and the simple Boolean terms were “MicroRNA,” “Glioma,” and “Glioblastoma.” For this analysis, only published studies were taken into consideration with the criteria being (a) studies in English, (b) studies on glioma and glioblastoma patients, (c) studies focusing on microRNA with either a tumor suppressing or inhibiting role or microRNA with tumor promoting action. Studies were excluded if they did not match this criterion or if they were (1) reviews or letters, (2) did not include any measurement for survival of patients, (3) had overlapping, duplicate, or insufficient data for the purpose of our analysis.
Quality Assessment
For the purpose of ensuring quality of the studies included in our analysis, a reviewer assessed the studies in accordance with critical review checklist of the Dutch Cochrane Centre proposed by MOOSE9 the checklist included (1) study population size, (2) type of carcinoma, (3) study design, (4) outcome assessment, (5) sufficient follow-up time, (6) microRNA being studied, (7) method to check microRNA level, and (8) microRNA cutoff value. A study was excluded from our analysis if it failed to provide anyone of the information listed above as to not compromise the validity of our meta-analysis. All the values were double checked by another reviewer to make sure no error occurred during the analysis.
Data Extraction
Data were extracted by a reviewer and then double checked by another reviewer to rule out any discrepancy or errors. The data extracted from the selected studies was as follows: the first author, year of publication, tumor and sample type, grade of tumor, sample size, duration of follow-up, microRNA being studied, method of testing microRNA, cutoff value for the microRNA, hazard ratio (HR) of microRNA as well as the 95% confidence interval and the p-value. In cases where the HR was not provided but the survival was given in terms of Kaplan–Meier survival curve or an appropriate summary statistic, the HR and its associated 95% confidence interval were calculated using the method described by Tierney et al.10
Statistical Analysis
For the purpose of our study, a fixed-effect model (Cochran-Mantel-Haenszel method) or the random effect model (DerSimonian–Laird method) was under consideration in accordance with the heterogeneity of the studies included. Using the hazard risks pooled from our studies and standard error for the HR calculated using the 95% confidence interval, the heterogeneity was verified using Higgins I2 statistic; in cases of heterogeneity being significant with p < 0.1 or I2 > 50%, a random effect model was used for our analysis. If not, then a fixed effect model was utilized. To minimize the influence of heterogeneity, the included studies were further subdivided to carry out subgroup analysis with studies having similar characteristics. To estimate publication bias in our study, Begg's and Egger's tests were performed using funnel plot with log of HR and standard error. In our study, p-values were calculated using a two-tailed test with p-value < 0.05 being statistically significant. To carry out all the statistical analysis, Stata16 (StataCorp LP, College Station, Texas, United States) software and Microsoft Excel (V.2010, Microsoft Corporation, Redmond, Washington, United States) program were used.
Results
Study Characteristics
A thorough and extensive literature search was performed by the method described in search strategy with 1082 studies being found to be of a potential interest. After the filtration and quality assessment steps mentioned in Fig. 1, a total of 20 articles were selected to be included in our meta-analysis. These studies included a total of 2262 patients with their prognosis and survival being compared with the level of microRNA. Out of these 20 studies, 9 were done on 11 microRNAs with tumor promoting action, while 11 of the articles were on 11 microRNA with a tumor suppressive action.
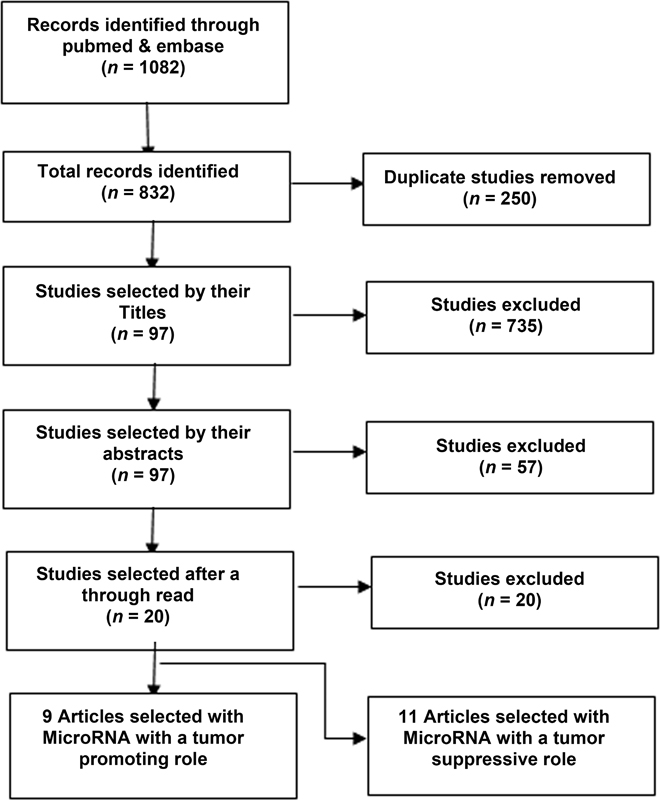
-
Fig. 1 Flowchart showing selection of articles for meta-analysis.
Correlation between Overall Survival and Expression of Tumor Promoting MicroRNA in Glioma
Random effect model was chosen for the analysis of the 11 tumor promoting microRNAs that have been shown in Table 1.11,12,13,14,15,16,17,18,19 The reason for this was the significant heterogeneity among our selected variable (I2 = 0.00%). The pooled HR for the overall survival and higher microRNA level was found to be 2.47 (95% CI = 1.98–2.96) as seen in Fig. 2, which shows the forest plot for our analysis. After performing a sensitivity analysis, it was seen that none of the included studies caused a significant alteration in the HR when removed.
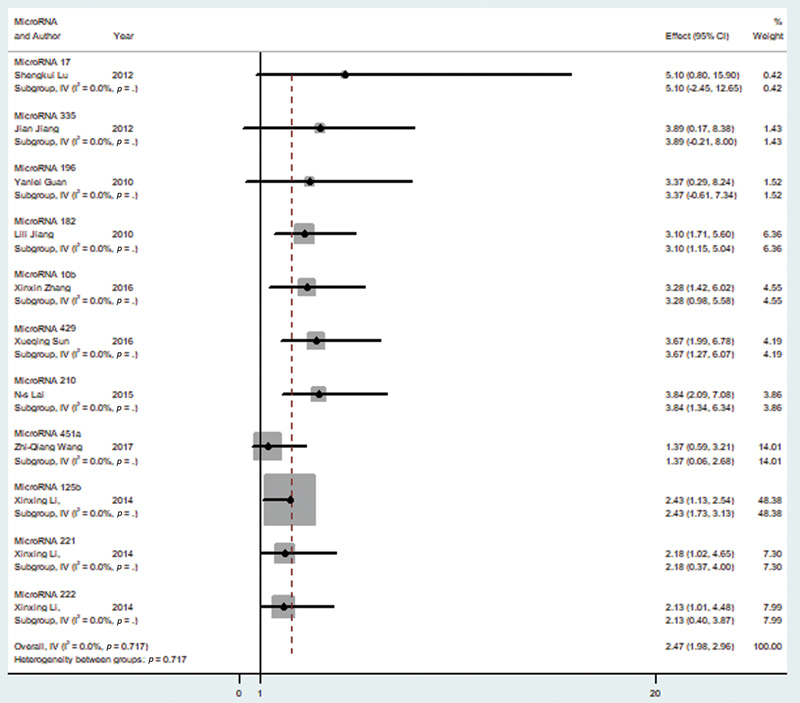
-
Fig. 2 Forest plot showing microRNA expression of tumor promoting microRNA and overall survival in glioma patients. CI, confidence interval.
Author |
Year |
Disease stage |
Sample type |
MicroRNA |
Method |
Sample size |
Cutoff |
Survival analysis |
Follow-up (months) |
Hazard ratio |
---|---|---|---|---|---|---|---|---|---|---|
Lu et al11 |
2012 |
I–IV |
Tissue |
MicroRNA 17 |
qRT-PCR |
108 |
Mean |
OS |
60 |
5.1 |
Jiang et al12 |
2012 |
I–IV |
Tissue |
MicroRNA 335 |
qRT-PCR |
166 |
Mean |
OS |
24 |
3.89 |
Guan et al13 |
2010 |
IV |
Tissue |
MicroRNA 196 |
qRT-PCR |
39 |
Median |
OS |
24 |
3.37 |
Jiang et al14 |
2010 |
I–IV |
Tissue |
MicroRNA 182 |
qRT-PCR |
253 |
Median |
OS |
60 |
3.1 |
Zhang et al15 |
2016 |
I–IV |
Tissue |
MicroRNA 10b |
qRT-PCR |
128 |
Median |
OS |
60 |
3.28 |
Sun et al16 |
2016 |
I–IV |
Tissue |
MicroRNA 429 |
qRT-PCR |
92 |
Mean |
OS |
72 |
3.67 |
Lai et al17 |
2015 |
I–IV |
Serum |
MicroRNA 210 |
qRT-PCR |
136 |
Median |
OS |
60 |
3.84 |
Wang et al18 |
2017 |
IV |
Serum |
MicroRNA 451a |
qRT-PCR |
48 |
Mean |
OS |
44 |
1.37 |
Li et al19 |
2014 |
I–IV |
Tissue |
MicroRNA 125b |
qRT-PCR |
45 |
Mean |
OS |
36 |
2.43 |
Li et al19 |
2014 |
I–IV |
Tissue |
MicroRNA 221 |
qRT-PCR |
45 |
Mean |
OS |
36 |
2.18 |
Li et al19 |
2014 |
I–IV |
Tissue |
MicroRNA 222 |
qRT-PCR |
45 |
Mean |
OS |
36 |
2.13 |
Abbreviations: OS, overall survival; qRT-PCR, quantitative reverse transcription polymerase chain reaction.
Further subgroup analysis was performed to compare pooled HR of each subgroup.
Subgroup Analysis
Subgroup analysis was as shown in Table 2. The studies were divided according to the tumor grade selected for the study, the cutoff value used, and the sample type selected for the study. In all the studies, it was seen that a higher microRNA level was associated with a higher WHO tumor grading in the studies that focused on grade I to IV (HR = 2.31; CI = 1.98–2.64) as well as those focusing on grade IV (HR = 2.82; CI = 2.35–3.29). The overall survival had a significant correlation with higher microRNA expression in both cases when the cutoff was taken as mean (HR = 2.53; CI = 2.41–2.64) or as median (HR = 3.21; CI: 2.80–3.62). Similar results for overall survival and high microRNA expression were found when the sample was collected from serum (HR = 2.52; CI = 2.11–2.93) or by tissue biopsy (HR = 2.61; CI = 2.21–3.01). The expression level of these microRNA was found to be high in patients with low Karnofsky performance score (KPS) as compared with those with a higher KPS (HR = 5.63; CI =: –3.12–14.38).
Subgroup analysis |
No. of studies |
No. of patients |
Model |
Pooled HR (95% CI) |
p-Value |
Heterogeneity (I2), p-Value |
---|---|---|---|---|---|---|
Grade I–IV |
7 |
928 |
Random |
2.31 (1.98–2.64) |
<0.001 |
81.4%, <0.001 |
Grade IV |
2 |
87 |
Fixed |
2.82 (2.35–3.29) |
<0.001 |
0.00%, 0.806 |
Cutoff value |
||||||
Mean |
5 |
459 |
Random |
2.53 (2.41–2.64) |
<0.001 |
83.4%, <0.001 |
Median |
4 |
556 |
Fixed |
3.21 (2.80–3.62) |
<0.001 |
0.00%, 0.842 |
Sample type |
||||||
Tissue |
7 |
831 |
Random |
2.61 (2.21–3.01) |
<0.001 |
79.6%, <0.001 |
Serum |
2 |
184 |
Fixed |
2.52 (2.11–2.93) |
<0.001 |
0.00%, 0.821 |
KPS (70 < vs. ≥ 70) |
4 |
547 |
Random |
5.63 (-3.12–14.38) |
<0.001 |
72.4%, <0.001 |
Abbreviations: CI, confidence interval; HR, hazard ratio; KPS, Karnofsky performance score.
Publication Bias
Publication bias in our study was assessed using the funnel plot analysis. As seen by Fig. 3, no significant publication bias was observed. Furthermore, the p-value for Egger's test was found to be 0.134 and for Begg's test, it was found to be 0.178 showing that there was no publication bias in our analysis.

-
Fig. 3 Funnel plot with pseudo 95 confidence interval for tumor promoting microRNA. HR, hazard ratio.
Correlation between Overall Survival and Expression of Tumor Suppressive MicroRNA in Glioma
The 11 studies selected for the purpose of this study20,21,22,23,24,25,26,27,28,29,30 as seen in Table 3 underwent random effect model for the overall survival analysis. This was due to significant heterogeneity being observed in the selected studies (p < 0.05, I2 = 85.9%). After carrying out the analysis, it was found that pooled HR for the overall survival and lower microRNA levels for our selected microRNA was 2.63 (95% CI = 2.44–2.81, p < 0.01). This can be seen in Fig. 4, showing forest plot for our analysis. Furthermore, a sensitivity analysis was performed to see if any study had a significant influence on our pooled HR. However, no significant alteration could be observed in the pooled HR following exclusion of each study. This showed that no particular study had a greater influence for the purpose of our analysis.
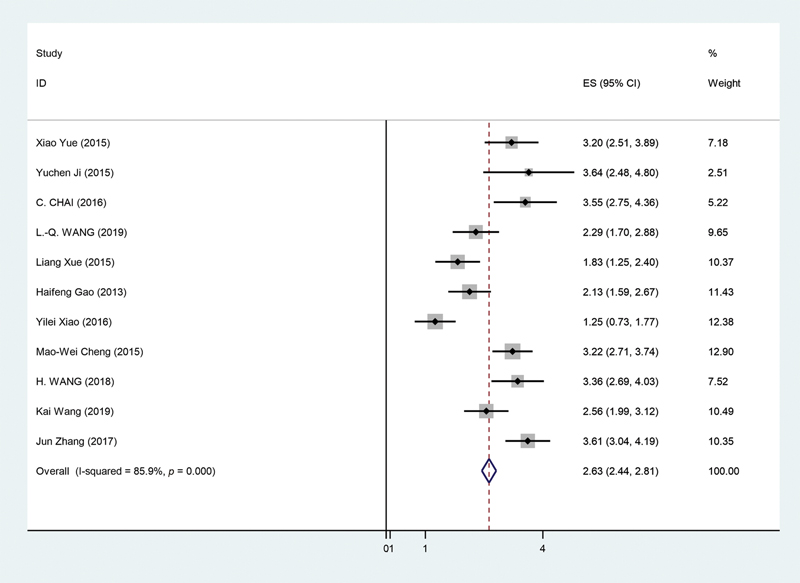
-
Fig. 4 Forest plot showing microRNA expression of tumor suppressive microRNA and overall survival in glioma patients. CI, confidence interval.
Author |
Year |
Disease stage |
Sample type |
MicroRNA |
Method |
Cutoff |
Survival analysis |
Follow-up (months) |
Hazard ratio |
---|---|---|---|---|---|---|---|---|---|
Yue et al20 |
2015 |
I–IV |
Serum |
MicroRNA-205 |
qRT-PCR |
Mean |
OS |
24 |
3.2 |
Ji et al22 |
2015 |
I–IV |
Tissue |
MicroRNA-107 |
qRT-PCR |
Mean |
OS |
60 |
3.64 |
Chai et al22 |
2016 |
I–IV |
Tissue |
MicroRNA-199a-3p |
qRT-PCR |
Mean |
OS |
24 |
3.552 |
Wang et al23 |
2019 |
I–IV |
Serum |
MicroRNA-124 |
qRT-PCR |
Mean |
OS |
15 |
2.289 |
Xue et al24 |
2015 |
II–IV |
Tissue |
MicroRNA-149 |
qRT-PCR |
Mean |
OS |
60 |
1.825 |
Gao et al25 |
2013 |
II–IV |
Tissue |
MicroRNA-34a |
qRT-PCR |
Median |
OS |
72 |
2.129 |
Xiao et al26 |
2016 |
II–IV |
Serum |
MicroRNA-182 |
qRT-PCR |
Mean |
OS |
36 |
1.25 |
Cheng et al27 |
2015 |
I–IV |
Tissue |
MicroRNA-218 |
qRT-PCR |
Mean |
OS |
60 |
3.225 |
Wang et al28 |
2018 |
I–IV |
Tissue |
MicroRNA-1231 |
qRT-PCR |
Mean |
OS |
60 |
3.356 |
Wang et al29 |
2019 |
I–IV |
Serum |
MicroRNA-769–3p |
qRT-PCR |
Mean |
OS |
60 |
2.556 |
Zhang et al30 |
2017 |
I–IV |
Tissue |
MicroRNA-211 |
qRT-PCR |
Mean |
OS |
60 |
3.614 |
Abbreviation: qRT-PCR, quantitative reverse transcription polymerase chain reaction.
Since significant heterogeneity was observed in our selected studies, subgroup analysis was performed to compare the pooled HR of each subgroup.
Subgroup Analysis
Subgroup analysis was performed to get a better understanding of our findings. The studies were divided according to the tumor grade selected for the study, the cutoff value used, and the sample type selected for the study. In all the studies, it was seen that a lower microRNA level was associated with a higher WHO tumor grading in the studies that focused on grade I/II versus III/IV (HR = 3.1; CI = 2.87–3.32) as well as those focusing on grade II versus III/IV (HR = 1.72; CI = 1.40–2.03). The overall survival had a significant correlation with lower microRNA expression in both cases when the cutoff was taken as mean (HR = 2.71; CI = 2.41–2.93) or as median (HR = 2.44; CI = 2.11–2.76). Similar results for overall survival and low microRNA expression were found when the sample was collected from serum (HR = 2.2; CI = 1.91–2.49) or by tissue biopsy (HR = 2.91; CI = 2.67–3.14). The expression level of microRNA was found to be high in patients with high KPS as compared with those with a lower KPS (HR = 7.53; CI = –13.47–28.54). Table 4 provides a summary of the subgroup analysis performed.
Subgroup analysis |
No. of studies |
No. of patients |
Model |
Pooled HR (95% CI) |
p-Value |
Heterogeneity (I2), p-Value |
---|---|---|---|---|---|---|
Grade I/II vs. III/IV |
8 |
821 |
Random |
3.1 (2.87–3.32) |
0.021 |
78.6%, 0.019 |
Grade II vs. III/IV |
3 |
426 |
Fixed |
1.72 (1.40–2.03) |
0.007 |
0.00%, 0.806 |
Cutoff value |
||||||
Mean |
7 |
699 |
Random |
2.71 (2.41–2.93) |
<0.001 |
88.4% <0.001 |
Median |
4 |
548 |
Fixed |
2.44 (2.11–2.76) |
<0.001 |
0.00%, 0.714 |
Sample type |
||||||
Tissue |
7 |
894 |
Random |
2.91 (2.67–3.14) |
<0.001 |
82.7%, <0.001 |
Serum |
4 |
353 |
Fixed |
2.2 (1.91–2.49) |
<0.001 |
0.00% 0.739 |
KPS (70 < vs. ≥ 70) |
5 |
561 |
Random |
7.53 (-13.47–28.54) |
<0.001 |
65.3%, <0.001 |
Abbreviations: CI, confidence interval; HR, hazard ratio; KPS, Karnofsky performance score.
As all these studies were done on Asian population and none on non-Asian population could be found that would fit our criteria for study selection, the difference in overall survival in different populations could not be calculated. Furthermore, the changes in microRNA expression in patients undergoing different therapies could not be observed as the selected studies did not mention it.
Publication Bias
Publication bias in our study was assessed using the funnel plot analysis. As seen by Fig. 5, no significant publication bias was observed. Furthermore, the p-value for Egger's test was found to be 0.194 and for Begg's test, it was found to be 0.206 showing that there was no publication bias in our analysis.
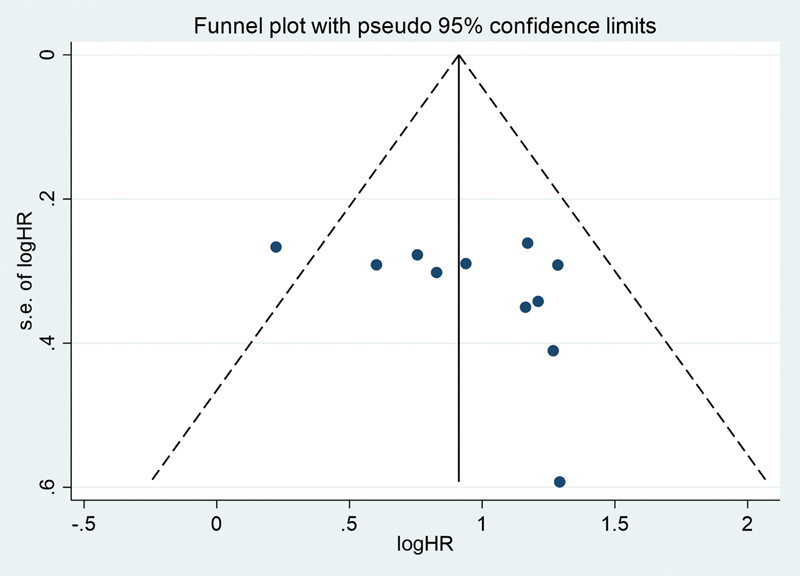
-
Fig. 5 Funnel plot with pseudo 95 confidence interval of tumor suppressive microRNA. HR, hazard ratio.
Discussion
The microRNA included in our studies not only affect the progression of glioma but of various other tumor throughout the body with their effects not only being limited to the brain. This suggests the possibility of their application in prognosis and management of a large number of tumors. Several studies have been performed discussing their role in different tumors. A summary of the mechanism of different microRNA and the tumors they affect is provided in Tables 5 and 6.
MicroRNA |
Other cancers |
Mechanism of tumor promotion in glioma |
---|---|---|
MicroRNA 17 |
Breast cancer, lung cancer, gastric cancer, colon cancer |
It has been found to target family of transcription factors like E2F and apoptotic proteins like BIM36,37,38 |
MicroRNA 335 |
Osteosarcoma, hepatocellular carcinoma, head and neck squamous cell carcinomas (HNSCC), renal cell carcinoma |
It targets Rb1 signaling pathway resulting in loss of control over cell cycle arrest in G0/G1 phase39 |
MicroRNA 196 |
Cervical, ovarian, pancreatic, and esophageal cancer |
It suppresses the expression of annexin A1 (a mediator of apoptosis) resulting in reduced apoptosis and rapid cell proliferation13 |
MicroRNA 182 |
Melanoma, papillary thyroid, and ovarian Cancer |
It reduces the function of FBXW7, which acts to induce apoptosis in cells via ubiquitination mediated degradation40,41 |
MicroRNA 10b |
Breast, hepatic, pancreatic, and esophageal cancer |
The downstream target of Mir-10b is Sirt1, which acts on the PTEN/PI3K/AKT signaling pathway to enhances growth of tumors42 |
MicroRNA 429 |
Ovarian cancer |
It targets c-myc and PLGG1 to exert its effects in several tumors43 |
MicroRNA 210 |
Tumor suppressor in esophageal cancer |
MicroRNA 210 is known to target to FBXO31 and plays a role in epithelial mesenchymal transition and Wnt/β-catenin signaling31 |
MicroRNA 451a |
Downregulated in small cell lung cancer and colon cancer |
The upregulation of microRNA 451a can reduce the action of BAP31 by binding to its 5′UTR. This gene also has an inhibitory role in myogenesis by inhibiting the C2C12 differentiation.32,44 |
MicroRNA 125b |
Glioma |
It regulates p53 gene and downregulation of mRNA 125b activates p38MAPK apoptotic pathway.45 |
MicroRNA 221/222 |
Glioma |
In cells with 221/222 gene in effect the bcl-2 is downregulated, while, connexin43, p27, PUMA, caspase-3, PTEN, TIMP3 and Bax is up-regulated46 |
MicroRNA |
Other cancers |
Mechanism of tumor suppression in glioma |
---|---|---|
MicroRNA-205 |
Lung cancer, ovarian cancer, bladder cancer, head and neck cancer |
Suppresses epithelial–mesenchymal transition and inhibits tumor growth of human glioma through down-regulation of HOXD947,48 |
MicroRNA-107 |
Bladder cancer, gastric cancer |
Acts via CDK6 signaling pathway and notch 2 modulation49 |
MicroRNA-199a-3p |
Renal carcinoma, bladder cancer, hepatocellular carcinoma |
Tumor suppression occurs via acting on the AKT/mTOR signaling pathway50,51 |
MicroRNA-218 |
cervical cancer, lung carcinoma, bladder cancer, gastric cancer |
Negatively affect the migration and invasiveness of gliomas by targeting NF-kB52,53 |
MicroRNA-1231 |
Nonsmall cell lung cancer |
Inhibits glioma progression both in vivo and in vitro by regulation of EGFR/PI3K/AKT pathway54 |
MicroRNA-211 |
Colorectal carcinoma, oral carcinoma, hepatocellular carcinoma, cervical cancer |
It targets MMP9, activates the caspase-9/caspase-3 apoptotic cascade, and was found to be hyper methylated in GB55,56 |
MicroRNA-124 |
Head and neck cancer, breast cancer |
|
MicroRNA-149 |
Nasopharyngeal carcinoma, melanoma, hepatocellular carcinoma, colorectal cancer, medullary carcinoma |
|
MicroRNA-34a |
Hepatocellular carcinoma, prostate cancer |
Targets c-Met and notch pathway62 |
MicroRNA-769–3p |
Melanoma, colorectal cancer |
Acts on Wnt/β-catenin pathway to inhibit the progression, migration, and invasion of cells63,64 |
To our knowledge, this meta-analysis is the first systemic evaluation of the relationship between the levels of several tumor suppressive and promoting microRNA and the prognosis of patients with glioma. Although there have been individual studies performed on the included microRNAs not only in glioma but also in tumors affecting other parts of the body as well, the prognostic potential of microRNAs in glioma is still unknown. Our study identified that a higher level of tumor promoting microRNA in a patient was predictive of poor survival (HR = 2.47; 95% CI = 1.98–2.96). Similarly, it was seen that a lower level of tumor suppressive microRNA was also associated with a poorer survival (HR = 2.63; 95% CI = 2.44–2.81, p < 0.01)). Moreover, both tumor promoting and suppressing microRNA were shown to have a strong association with the WHO grade of the tumor.
Recently several studies have proved that microRNA associated with tumor promoting role in one malignancy can be playing a tumor suppressive role in another. For example, microRNA 210 and 451a that despite being oncogenic in the case of glioma have shown to downregulate cancer cell in esophageal cancer and small cell carcinoma of the lung, respectively.31,32 As seen in Tables 5 and 6, all of these microRNAs are involved in pathogenesis of various tumors and thus it shows that their application as a prognostic tool would not only be limited to glioma.
Several studies have already discussed in detail how microRNA can be a prognostic tool for prediction of survival in various tumor. A meta-analysis done by Li et al showed the prognostic potential of microRNA 21 in glioma patients.33 Similarly, another meta-analysis discussed the role microRNA 34a could play in prognosis of gastric cancer.34 A review by Lee and Dutta not only discussed the prognostic role of microRNAs in tumor but also the possible role they could play in cancer therapy.35 In our meta-analysis, it was seen that both tumor suppressive and promoting microRNAs could be used as prognostic tools; moreover, in the subgroup analysis it was seen that the levels of microRNA obtained from both tumor biopsy and from serum had a clinically significant association with survival thus making liquid biopsy to predict tumor prognosis a possibility. Such a technique would be especially useful in less developed countries where performing a biopsy might not always be an option. It would also have an additional advantage of being less invasive and cheaper.
Limitations
At present, our study has some limitations, one of which is the heterogeneity in the results, which is mainly due to the small number of studies, the difference in microRNAs selected, and the variation in their cutoff value. As seen above, some of the studies used mean cutoff values while some used median. Due to these reasons, a random effect model was used in our analysis. Furthermore, the only studies that could be included in the analysis were done on Chinese patients so no comparison could be made with other ethnicities.
Due to a relatively small sample size of glioma patients, the statistical power of the association between microRNA level and glioma is reduced. Also, different microRNA being included with no standard cutoff value further contributes to bias and may deviate the pooled results from accurate findings. Furthermore, there is heterogeneity between overall survival analysis that can be associated with different stage of disease as well as different microRNAs were included; to counter this, future studies would be needed to study each individual microRNA in more detail.
Despite these limitations, the evidence above is sufficient to say with credibility that these microRNA do have possibility to be used as prognosis markers.
Conclusion
As evidenced by our analysis, it can be seen that all of our microRNAs selected could be used as potential biomarkers for glioma. However, still further studies are needed to explore their future applications. Prospective studies, which should be multicentric with a large sample size, would still be needed to confirm the validity of the results. Studies in clinical setting focusing on prognostic value of these microRNAs done on different population would further be needed. For now, it can be concluded that these microRNA do play a role in tumor pathogenesis and their expression level could be used as indicator of survival and disease prognosis.
Conflict of Interest
None declared.
Funding None.
References
- The role of molecular diagnostics in the management of patients with gliomas. Current treatment options in oncology. 2016;17(10):1-6.
- [Google Scholar]
- Origin of gliomas Thieme Medical Publishers; InSeminars in neurology 2018 Feb (Vol. 38, No. 01, pp. 005-010).
- [Google Scholar]
- Molecular Classification of Gliomas. Vol. 134, Handbook of Clinical Neurology Handbook of Clinical Neurology; 2016. p. :97-120.
- [Google Scholar]
- Overview of microRNA biogenesis, mechanisms of actions, and circulation Vol 9. Frontiers in endocrinology; 2018. p. :402.
- [Google Scholar]
- MicroRNAs in cancer treatment and prognosis. Am J Cancer Res. 2012;2(04):414-433. http://www.ncbi.nlm.nih.gov/pubmed/22860232 cited2020Aug26 [Internet]
- [Google Scholar]
- Meta-analysis of observational studies in epidemiology: a proposal for reporting. Meta-analysis Of Observational Studies in Epidemiology (MOOSE) group. JAMA. 2000;283(15):2008-2012. [Internet]
- [CrossRef] [Google Scholar]
- Practical methods for incorporating summary time-to-event data into meta-analysis. Trials. 2007;8(01):1-6.
- [Google Scholar]
- Increased expression of microRNA-17 predicts poor prognosis in human glioma. J Biomed Biotechnol. 2012;2012:970761.
- [Google Scholar]
- Tumor microRNA-335 expression is associated with poor prognosis in human glioma. Med Oncol. 2012;29(05):3472-3477.
- [Google Scholar]
- MiRNA-196 is upregulated in glioblastoma but not in anaplastic astrocytoma and has prognostic significance. Clin Cancer Res. 2010;16(16):4289-4297.
- [Google Scholar]
- miR-182 as a prognostic marker for glioma progression and patient survival. Am J Pathol. 2010;177(01):29-38.
- [Google Scholar]
- Overexpression of tissue microRNA10b may help predict glioma prognosis. J Clin Neurosci. 2016;29:59-63.
- [Google Scholar]
- The potential prognostic value of microRNA-429 for human gliomas. Ann Clin Lab Sci. 2016;46(01):44-48.
- [Google Scholar]
- Serum microRNA-210 as a potential noninvasive biomarker for the diagnosis and prognosis of glioma. Br J Cancer. 2015;112(07):1241-1246.
- [Google Scholar]
- Low serum level of miR-485-3p predicts poor survival in patients with glioblastoma. PLoS One. 2017;12(09):e0184969.
- [Google Scholar]
- Predictive and prognostic roles of abnormal expression of tissue miR-125b, miR-221, and miR-222 in glioma. Mol Neurobiol. 2016;53(01):577-583.
- [Google Scholar]
- Downregulation of serum microRNA-205 as a potential diagnostic and prognostic biomarker for human glioma. J Neurosurg. 2016;124(01):122-128.
- [Google Scholar]
- Decreased expression of microRNA-107 predicts poorer prognosis in glioma. Tumour Biol. 2015;36(06):4461-4466.
- [Google Scholar]
- Circulating miR-199a-3p in plasma and its potential diagnostic and prognostic value in glioma. Eur Rev Med Pharmacol Sci. 2016;20(23):4885-4890.
- [Google Scholar]
- Downregulation of plasma miR-124 expression is a predictive biomarker for prognosis of glioma. Eur Rev Med Pharmacol Sci. 2019;23(01):271-276.
- [Google Scholar]
- Low MiR-149 expression is associated with unfavorable prognosis and enhanced Akt/mTOR signaling in glioma. Int J Clin Exp Pathol. 2015;8(09):11178-11184.
- [Google Scholar]
- Expression level of human miR-34a correlates with glioma grade and prognosis. J Neurooncol. 2013;113(02):221-228.
- [Google Scholar]
- Potential diagnostic and prognostic value of plasma circulating microrna-182 in human glioma. Med Sci Monit. 2016;22:855-862.
- [Google Scholar]
- Expression of microRNA-218 and its clinicopathological and prognostic significance in human glioma cases. Asian Pac J Cancer Prev. 2015;16(05):1839-1843.
- [Google Scholar]
- Low expression of miR-1231 in patients with glioma and its prognostic significance. Eur Rev Med Pharmacol Sci. 2018;22(23):8399-8405.
- [Google Scholar]
- MicroRNA-769-3p inhibits tumor progression in glioma by suppressing ZEB2 and inhibiting the Wnt/β-catenin signaling pathway. Oncol Lett. 2020;19(01):992-1000.
- [Google Scholar]
- MicroRNA-211 expression is down-regulated and associated with poor prognosis in human glioma. J Neurooncol. 2017;133(03):553-559.
- [Google Scholar]
- MicroRNA-210 targets FBXO31 to inhibit tumor progression and regulates the Wnt/β-catenin signaling pathway and EMT in esophageal squamous cell carcinoma. Thorac Cancer. 2021;12(06):932-940.
- [Google Scholar]
- Loss of miR-451a enhances SPARC production during myogenesis. PLoS One. 2019;14(03):e0214301.
- [CrossRef] [Google Scholar]
- Prognostic role of microRNA-21 expression in gliomas: a meta-analysis. J Neurooncol. 2016;130(01):11-17.
- [Google Scholar]
- A meta-analysis of the effect of microRNA-34a on the progression and prognosis of gastric cancer. Eur Rev Med Pharmacol Sci. 2018;22(23):8281-8287.
- [Google Scholar]
- Clinical significance of MicroRNA expression profiles and polymorphisms in lung cancer development and management. Pathol Res Int. 2011;2011:780652.
- [Google Scholar]
- Antagomir-17-5p abolishes the growth of therapy-resistant neuroblastoma through p21 and BIM. PLoS One. 2008;3(05):e2236.
- [Google Scholar]
- A microRNA expression signature of human solid tumors defines cancer gene targets. Proc Natl Acad Sci U S A. 2006;103(07):2257-2261.
- [Google Scholar]
- Identification of hsa-miR-335 as a prognostic signature in gastric cancer. PLoS One. 2012;7(07):e40037.
- [Google Scholar]
- Roles of miR-182 in sensory organ development and cancer. Thorac Cancer. 2015;6(01):2-9.
- [Google Scholar]
- MiR-182 promotes glioma progression by targeting FBXW7. J Neurol Sci. 2020;411:116689.
- [Google Scholar]
- SIRT1 promotes tumorigenesis of hepatocellular carcinoma through PI3K/PTEN/AKT signaling. Oncol Rep. 2012;28(01):311-318.
- [Google Scholar]
- miR-429 inhibits glioma invasion through BMK1 suppression. J Neurooncol. 2015;125(01):43-54.
- [Google Scholar]
- MiR-451a suppressing BAP31 can inhibit proliferation and increase apoptosis through inducing ER stress in colorectal cancer. Cell Death Dis. 2019;10(03):152.
- [CrossRef] [Google Scholar]
- MiR-125b acts as an oncogene in glioblastoma cells and inhibits cell apoptosis through p53 and p38MAPK-independent pathways. Br J Cancer. 2013;109(11):2853-2863.
- [Google Scholar]
- [Inhibitory effect of knocking down microRNA-221 and microRNA-222 on glioma cell growth in vitro and in vivo] Zhonghua Zhong Liu Za Zhi. 2009;31(10):721-726.
- [Google Scholar]
- Micro-RNA profiling in kidney and bladder cancers. InUrologic Oncology: Seminars and Original Investigations 2007 Sep 1 (Vol. 25, No. 5, pp. 387-392) Elsevier;
- [Google Scholar]
- MiR-205 suppresses epithelial-mesenchymal transition and inhibits tumor growth of human glioma through down-regulation of HOXD9. Biosci Rep. 2019;39(05):39.
- [Google Scholar]
- P53-induced microRNA-107 inhibits proliferation of glioma cells and down-regulates the expression of CDK6 and Notch-2. Neurosci Lett. 2013;534:327-332.
- [Google Scholar]
- MicroRNA-199a-3p suppresses glioma cell proliferation by regulating the AKT/mTOR signaling pathway. Tumour Biol. 2015;36(09):6929-6938.
- [Google Scholar]
- miR-199a-3p inhibits cell proliferation and induces apoptosis by targeting YAP1, suppressing Jagged1-Notch signaling in human hepatocellular carcinoma. J Biomed Sci. 2016;23(01):79.
- [Google Scholar]
- miR-218 inhibits the invasive ability of glioma cells by direct downregulation of IKK-β. Biochem Biophys Res Commun. 2010;402(01):135-140.
- [Google Scholar]
- MicroRNA-218 functions as a tumor suppressor in lung cancer by targeting IL-6/STAT3 and negatively correlates with poor prognosis. Mol Cancer. 2017;16(01):141.
- [Google Scholar]
- MicroRNA-1231 exerts a tumor suppressor role through regulating the EGFR/PI3K/AKT axis in glioma. J Neurooncol. 2018;139(03):547-562.
- [Google Scholar]
- Association between high miR-211 microRNA expression and the poor prognosis of oral carcinoma. J Dent Res. 2008;87(11):1063-1068.
- [Google Scholar]
- Downregulation of microRNA-124 predicts poor prognosis in glioma patients. Neurol Sci. 2015;36(01):131-135.
- [Google Scholar]
- MicroRNA-124 inhibits the proliferation of C6 glioma cells by targeting Smad4. Int J Mol Med. 2017;40(04):1226-1234.
- [Google Scholar]
- Comprehensive analysis of microRNA-regulated protein interaction network reveals the tumor suppressive role of microRNA-149 in human hepatocellular carcinoma via targeting AKT-mTOR pathway. Mol Cancer. 2014;13:253.
- [Google Scholar]
- MicroRNA-149 suppresses colorectal cancer cell migration and invasion by directly targeting forkhead box transcription factor FOXM1. Cell Physiol Biochem. 2015;35(02):499-515.
- [Google Scholar]
- miR-149-5p inhibits cell proliferation and invasion through targeting GIT1 in medullary thyroid carcinoma. Oncol Lett. 2019;17(01):372-378.
- [Google Scholar]
- microRNA-34a is tumor suppressive in brain tumors and glioma stem cells. Cell Cycle. 2010;9(06):1031-1036.
- [Google Scholar]
- MiR-769 inhibits colorectal cancer cell proliferation and invasion by targeting HEY1. Med Sci Monit. 2018;24:9232-9239.
- [Google Scholar]
- Identification of aberrant microRNA expression pattern in pediatric gliomas by microarray. Diagn Pathol. 2013;8:158.
- [Google Scholar]
- MiRNA-196 is upregulated in glioblastoma but not in anaplastic astrocytoma and has prognostic significance. Clin Cancer Res. 2010;16(16):4289-4297.
- [Google Scholar]