Translate this page into:
Molecular oncology update: Breast cancer gene expression profiling
Address for correspondence: Dr. Amit Verma, Max Cancer Center, Max Hospital, New Delhi, India. amitverma.dr@gmail.com
This article was originally published by Thieme Medical and Scientific Publishers Private Ltd. and was migrated to Scientific Scholar after the change of Publisher.
Abstract
Molecular Oncology has paved its way in the comprehensive cancer care, and its burgeoning role especially in the discovery of novel cellular targets is responsible for emergence of the new Paradigm called “Genomic Paradigm”, a shift from the old age “Clinico-Pathological Paradigm”. In breast cancer, till recently clinical decisions, prognostications, and predictions were based solely upon histopathologic analysis, or one or a small numbers of genes or their expressions (proteins) in the tumor tissue. With the advent of newer technologies like microarray, which allows us to read the “Molecular Signature” of an individual patient's tumor, the molecular information is exploited for novel targeted treatments and new biomarkers identification. This has led to better classification of cancers for prognostication and treatment selection. In this review we have summarized various Genomic Expression Profiling (GEP) which are available commercially or in different developmental phases.
Keywords
Breast cancer
classification
gene expression
genomics
next generation sequencing
prognosis
Introduction
In the current era, molecular oncology has paved its way in the comprehensive cancer care and is playing an important role especially in the discovery of novel cellular targets to exploit for novel targeted treatments, new biomarkers identification for early cancer detection, and to provide a better classification of cancers for prognostication and treatment selection. The molecular basis of breast carcinogenesis involving genetic and epigenetic events that result in altered expression of numerous genes is yet not fully understood and still needs to be deciphered more, which reflects the complexity of the molecular alterations that characterize tumor cells.
Most of the breast cancer genome is selectively repressed; approximately, 3–5% of genes are active in a particular tumor cell that is governed by the regulation of gene expression, mostly at the level of transcription. Cellular perturbation (transformation) causes changes in gene expression that result in the expression of hundreds of gene products and the suppression of others. Thus, molecular heterogeneity is generated that characterizes tumors of different histology and contributes to the variability in outcome and response to therapy. Further, significant variability also exists for tumors of a specific histologic type. Till recently, in breast cancer management, clinical decisions, prognostications, and predictions were based solely upon histopathologic analysis, or one or small numbers of genes or their expressions (proteins) in the tumor tissue, but this approach has been inadequate and failed to generate accurate conclusions. There has been an emergence of the new paradigm called “Genomic Paradigm,” a shift from the old age “Clinico-Pathological Paradigm” Figure 1.
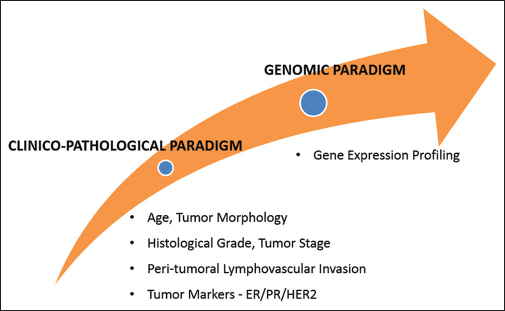
- Changing Genomic Paradigm
The examination of multiple expressed genes provides more useful information for both classification and prognostication of individual tumors. The microarray methodology, which permits the analysis and quantitation of the expression of thousands of genes simultaneously, is a powerful technique to read the “Molecular Signature” of an individual patient's tumor, and the process is termed gene expression profiling (GEP). Analyzing gene expression patterns across individual patients with the same disease may reveal molecular differences that may allow molecular subclassification, better treatment selection, and prognostication. The molecular heterogeneity of breast cancer that involves a large number of genes controlling cell growth, death, and differentiation warrants studying of multiple genetic alterations in concert to cater the patient in more precise and tailored way. Various GEPs are now available that allows the simultaneous measurement of the expression of thousands of genes in a breast cancer cell.
The current applications of the breast GEP are as follows:
-
Molecular subclassification
-
Prognostication
-
Prediction.
Molecular Subclassification (Intrinsic Subtypes)
Several distinct breast cancer subtypes have been identified that differ markedly in prognosis and in the therapeutic targets they express.1,2,3,4,5,6,7,8,9 The genes that identify these subtypes (intrinsic subtypes) are represented in various clusters, including gene cluster related to estrogen receptor (ER) expression (the luminal cluster), human epidermal growth factor 2 (HER2) expression, proliferation, and a unique cluster of genes called the basal cluster.
Luminal subtypes
Luminal A and luminal B express genes associated with luminal epithelial cells of normal breast tissue that typically express luminal cytokeratins 8 and 18. These are the most common subtypes and make up the majority of ER-positive breast cancer. The routine clinical assay (immunohistochemistry [IHC]) characterizes them by high expression of ER, progesterone receptor (PR), and other genes associated with ER activation. Further, the luminal A and luminal B subtypes have some important molecular and prognostic distinctions Table 1.
Luminal A |
Luminal B |
|
---|---|---|
Incidence (%) |
40 |
20 |
ER-related genes |
High |
Relatively low |
HER2-related genes |
Low |
Variable |
Proliferation-related genes |
Low |
High |
Prognostic significance |
Good |
Poor |
Prediction to endocrine Rx |
Highly sensitive |
Relatively less sensitive |
Prediction to cytotoxic Rx |
Less sensitive |
Less sensitive |
ER - Estrogen receptor; HER2 - Human epidermal growth factor 2
Human epidermal growth factor 2-enriched
The subtype represent up about 10–15% of breast cancers and is characterized by high expression of the HER2 and proliferation gene clusters, low expression of luminal clusters genes (CK-7, 8, 18, 19, X-box-binding protein 1, GATA-binding protein 3, hepatocyte nuclear factor 3, Annexin XXXI, and ER 1). These tumors are most often positive for HER2 and negative for ER and PR. About 30% of HER2-enriched tumors are clinically HER2-negative, and on the other, 50% of clinical HER2-positive breast cancers are HER2-enriched. They have a poorer prognosis compared with luminal A tumors and are predictive of response to herceptin.
Estrogen receptor-negative subtypes
ER-negative subtypes – this includes multiple subtypes, such as basal-like, claudin-low,1 and interferon-rich,10 molecular apocrine. The basal-like subtype has some similarity in gene expression to that of the basal epithelial cells of normal breast tissue (expressing CK-5, 6, 17, integrin-β4, laminin, and fatty-acid binding protein 7.4) and represent about 15–20% of breast cancers. It is characterized by low expression of the luminal and HER2 gene clusters thus similar to triple-negative breast cancers (TNBCs). However, there is a significant discordance (up to 30%) between the basal-like and TNBC. These subtypes exhibit high frequency of BRCA1 mutations, increased genomic instability, high histologic grade, and have a poorer prognosis compared with luminal A tumors.
Claudin-low breast cancer
Claudin-low breast cancer represents 5% of breast cancers and is characterized by overexpression of genes associated with epithelial-mesenchymal transition-like chemokine ligand 12, vimentin and fibroblast growth factor 7, Krüppel-like factor 2, integrin α5, moesin, vascular endothelial growth factor-C, matrix metalloproteinase-9, CD79b, CD14, CD44+/CD24−, and ALDH1A1. This subtype frequently exhibits metaplastic and medullary differentiation and are similar to TNBC with a poorer prognosis compared with luminal A tumors.
Interferon-rich and molecular apocrine subtypes
These subtypes are rare, characterized by interferon-regulated genes, such as STAT1 and activation of androgen receptor signaling, respectively.
Normal-like subtypes
Normal-like subtypes are typified by similar gene expression pattern as the normal breast. It yet remains enigmatic as to whether it represents a separate subtype or a technical artifact introduced by low tumor cell composition of the sampled specimen.
Tools for molecular classification
For identification of four-basal-like, HER2-enriched, luminal A and luminal B subtypes, tools called single sample predictors (SSPs) are available including Blueprint, SSP203 (500 genes), SSP2006 (306 genes), and predictor analysis of microarray 50 (PAM50) (50 genes).6,11,12 The SSPs utilizes large sets of tumor-intrinsic genes, which are analyzed by hierarchical clustering followed by nearest centroid classification. The limitation includes subtyping is dependent upon the type of SSP used for analysis, another intrinsic subtype called normal breast-like subtype is considered invalid and HER2 enriched subtype does not encompass all cases classified as HER2-positive by IHC/fluorescence in situ hybridization.
Prognosis
Molecular prognostic profiles are used to predict the local as well as distant recurrence rate in early breast cancers while receiving endocrine therapy and the absolute benefit of adjuvant chemotherapy to reduce the incidence of the recurrence. The most commonly used and commercially available molecular prognostic profiles are Oncotype DX (21-gene derived recurrence score [RS]), the MammaPrint (Amsterdam 70-gene derived RS), and PAM50 (50-gene derived RS).
Oncotype DX
The 21-gene RS is well-validated prognostic assays. The assay calculates RS viz. low (RS < 18), intermediate (RS 18–30), or high score (RS > 30) using mathematical formula that includes sum of expression of 16 cancer-related genes plus 5 reference genes as shown in Table 2, generated using reverse-transcriptase polymerase chain reaction (PCR) on the formalin-fixed, paraffin embedded (FFPE) blocks, which are optimized to predict the locoregional and distant relapse despite tamoxifen therapy. Further, RS is able to predict relapse with aromatase inhibitors in postmenopausal women. The RS was validated in various large, independent retrospective multicenter trials involving over 3300 patients. The key study includes NSABP study B-14, NSABP B-20, Kaiser Permanente study, Southwest Oncology Group (SWOG) 8814 study. Currently, prospective trials (i) Oncotype DX Trial Assigning IndividuaLized Options for Treatment (Rx) (TAILORx) trial and (ii) RxPONDER trial (SWOG S1007) are ongoing to validate the predictive potential of Oncotype DX.13,14,15,16,17,18,19,20
Genes category |
Genes |
|
---|---|---|
Cancer-related genes16 |
Proliferation |
Ki67, STK15, Survivin, CCNB1, MYBL2 |
Invasion |
MMP11. CTSL2 |
|
HER2 |
GRB2, HER2 |
|
Estrogen |
ER, PGR, BCL2, SCUBE2 |
|
Other cancer-related genes |
GSTM1, CD68, BAG1 |
|
Reference genes5 |
ACTB, GAPDH, RPLPO, GUS, TFRC |
HER2 - Human epidermal growth factor 2
Oncotype DX is included in guidelines-St Gallen, European Society for Medical Oncology, American Society of Clinical Oncology, National Comprehensive Cancer Network as a decision tool enabling the identification of patients who are most likely to benefit from adjuvant chemotherapy and is indicated for women with node-negative, ER-positive breast cancer to determine the prognosis in patients recommended proceeding with at least a 5-year course of endocrine therapy.
Amsterdam 70-gene profile
US Food and Drug Administration approved commercially available test, known as MammaPrint, uses microarray technology to analyze 70 genes extracted from either FFPE tissue or fresh frozen tissue. It is recommended for early breast cancer of all ages with tumors size <5 cm, with the lymph node involvement up to three nodes. Unlike Oncotype DX, it can be used to determine the prognosis in patients with breast cancer regardless of hormone receptor-status and in patients with the HER2 positive disease. MammaPrint categorizes patient into two groups (i) low risk (ii) and high risk group for breast cancer distant relapse within 10 years of the initial diagnosis.
The 70 genes can be classified into various categories as shown in Table 3.
Biological function |
MammaPrint gene count |
---|---|
Metabolism |
7 |
Cell cycle and DNA replication |
12 |
Extracellular matrix adhesion and remodeling |
5 |
Growth, proliferation, transformation, and cell death |
17 |
General signal transduction and intracellular transport |
3 |
Growth factor |
7 |
Motility or actin filament related |
5 |
Intracellular hydrolase |
1 |
Immune response |
1 |
Neuropeptide |
1 |
Predicted transmembrane protein |
2 |
Predicted transcriptional control or DNA binding protein |
5 |
Unknown |
4 |
Total |
70 |
The clinical validity of the 70-gene prognostic profile has been demonstrated in multiple studies, including retrospective analysis of 295 consecutive invasive breast tumors from the tumor bank at the Netherlands Cancer Institute, 302 patient data contributed by the TRANSBIG consortium (5 participating European hospitals), prospective analysis of 427 women in The MicroarRAy PrognoSTics in Breast CancER (RASTER) trial. Currently, a multicentric prospective The Microarray in Node negative and 1–3 positive lymph node Disease May Avoid ChemoTherapy (MINDACT) clinical trial is ongoing which includes 6000 patients from 93 participating institutions in nine European countries, the results of which are awaited.21,22,23,24,25,26,27,28,29
Predictor analysis of microarray 50 risk of recurrence score
The PAM50 is a 50-gene test utilizes microarray and quantitative reverse transcription PCR technology on FFPE tissue. The PAM50 test characterizes an individual tumor by intrinsic subtype and along with tumor size, generates the risk of recurrence (ROR) score that can stratify patients with ER-positive, node-negative disease into high, medium, and low subsets.11,30,31
The clinical utility of the PAM50 and ROR score has been demonstrated in several studies31,32,33,34 including ATAC trial (adjuvant tamoxifen or anastrazole), a retrospective analysis of 1017 postmenopausal patients and Austrian Breast Cancer Study Group 8 (ABCSG-8) trial, a retrospective analysis of 1478 postmenopausal patients, where ROR predicted the risk of distant recurrence at 10 years in both node-negative and node-positive disease and added significant prognostic information in all subgroups of patients.34,35
Other Assays
Rotterdam 76-gene signature
The Rotterdam/Veridex 76-gene prognostic signature uses separate prognostic gene sets for ER-negative (16 genes) and ER-positive (60 genes) disease.36 The 76 prognostic gene signature has been evaluated in an independent set of 171 tumors, demonstrating 93% sensitivity and 48% specificity predicting distant metastasis-free survival independent of clinical variables. Currently, the test is not commercially available and warrant more studies to validate the clinical utility of this test prior to its use in clinical practice.
Genomic grade index
Meant for improving prognostic value of tumor grading, which involves analysis of gene expression signature of 97 genes that distinguish histological Grade 1 from Grade 3 tumors37 and reclassify Grade 2 tumors into two groups of high versus low ROR.37 Subgroup analysis of 204 patients treated in the PACS01 trial, genomic grade index outperformed histologic grade and other proliferation markers (Ki67 messenger RNA and protein, mitotic activity index) as a predictor of disease-free survival.38 Further studies are required for its clinical utility.
Molecular grade index
Another tumor grading multigene assay reclassifies Grade 2 tumors into Grade 1-like and Grade 3-like tumors. It involves 5-gene expression index to stratify patients into low or high ROR.39
Breast cancer index
Breast cancer index (BCI) is a combination of two profiles, the HOXB13-to-IL17BR expression ratio (H: I ratio) and the molecular grade index. Various studies have validated the BCI as a predictor of endocrine responsiveness among patients with ER-positive breast cancer39 and of prognosis.39,40 Yet again, BCI clinical utility remains to be determined.
Endopredict
Involves RNA-based analysis of 11 genes (8 cancer related and 3 reference genes) to calculate a prognostic score. Its prognostic value is independent of conventional prognostic factors41 that was validated using the data from two ABCSG trials (ABCSG-6 and ABCSG-8). However, the performance of EP as compared with other prognostic tests has not been evaluated.
Limitation of the multigene assays
There is a lack of level I evidence. The evidence is based on the retrospective analysis; results of the two randomized prospective trials, MINDACT and TAILORx are still awaited. Based on the TAILORx interim analysis of the women in the lowest- risk group suggests that at 5 years, rates of distant relapse-free survival were 99.3 percent, of invasive disease free survival were 93.8 percent, and of overall survival were 98.0 percent. These results provide prospective evidence that the gene expression test identifies women with a low risk of recurrence who can be spared chemotherapy.19 The list of genes in various assays is not stable with negligible overlap but have similar performance and a good concordance. The bias of determining the prognosis correlates with the proliferation-/cell cycle-related genes, thus, multigene assay serves mere surrogates of proliferation. The application is limited for the ER-positive and HER2-negative breast cancers but cannot determine the prognosis for the early stage TNBC. Almost 40–60% of clinically intermediate-risk patients are found to be intermediate-risk RS group, thus the clinical dilemma where prognostication and management remains unclear. The prediction potential is valid for short-term distant recurrence (<5 years) but indeterminate prognostic potential after 5–10 years of follow-up. This multigene assay doesnot consider analysis of tumor tissue microenvironment including stromal cells, inflammatory infiltrate, and normal breast tissue. The percentage of nonneoplastic cells has a substantial impact on the final expression profile and meaningful prognostic signatures. Thus, next generation assays taking this analysis into account and overcoming the limitation are under development.
Second generation gene expression signatures
Most of the first generation signatures as discussed above were developed based on the epithelial cancer cells, and the contribution of stromal cells was not taken into account. The accuracy of gene predictors assay may be influenced by varying proportions of stromal components,42 and comprehensive GEP of each cell type has demonstrated that at the transcriptome level, changes occur in epithelial, myoepithelial, and stromal cells.43 Various research groups have used prognostic signatures derived from the profile of stromal cells,44,45,46 by analyzing the immune system44,47,48 or cancer-related pathways49,50,51 as shown in Table 4.
Category |
Gene signature (second generation) |
Signature development/rationale |
---|---|---|
Stroma signatures |
PLAU module44 |
PLAU represent a prototype gene set for tumor invasion/metastasis are analyzed along with other gene sets for different biological processes (ER for ER signaling, HER2 for HER2 signaling, AURKA for proliferation, CASP3 for apoptosis, STAT1 for immune response, VEGF for angiogenesis) |
SDPP45 |
A stroma-derived predictor using three genes clusters was developed after ranking the genes by their independent prognostic ability using a multivariate logistic regression with clinical and biological variables as covariates |
|
DCN module46 |
Decorin gene set is used representing stroma was selected and used as a variable in a multivariate regression model |
|
Immune-related signatures |
STAT1 module44 |
STAT1 represent a prototype gene set for immune response is analyzed along with other gene sets for different biological processes |
IR module47 |
A subclass of ER - tumors that over-express immune response genes are known to have good prognosis independent of lymph nodes status or lymphocytic infiltration are analyzed |
|
IgG, HCK, MHC-I, MHC-II, LCK, STAT1, interferon metagenes48 |
Seven metagenes were derived from unsupervised hierarchical clustering of genes in 12 primary invasive breast cancer datasets, where each metagenes is associated with a cell type and/or immunological state |
|
Pathways signatures |
PIK3CA-GS49 |
A PIK3CA mutation-associated gene signature developed based on the differentially expressed genes between PIK3CA mutant and wild-type ER+/HER2− breast cancers |
IGF-I gene signature50 |
IGF-I gene expression signatures were developed based on the differential gene expressions after treatment of MCF-7 breast cancer cell line with IGF-I |
|
MYC, RAS, E2F3, SRC, and b-CATENIN signatures51 |
Gene expression signatures that reflect the activity of a given pathway were identified that correlated with the classification of human primary mammary epithelial cell cultures samples into oncogene-activated/deregulated versus control |
ER - Estrogen receptor; HER2 - Human epidermal growth factor 2; VEGF - Vascular endothelial growth factor; IGF-I - Insulin-like growth factor-I
Prediction
In routine clinical practice, only ER and HER2 are used as predictive markers for the selection of patients likely to respond to endocrine therapy and trastuzumab, respectively. Multigene assay is currently being used/experimented to provide additional information that could predict the response or lack of response to treatments and determine the most effective regimen for a specific patient or subgroup of patients.
Multigene markers of response to chemotherapy
Oncotype DX-RS predicts the benefit from the addition of chemotherapy to tamoxifen. This was evident in NSABP B-20 trial where node-negative, ER-positive breast cancers were treated with Cyclophosphamide, Methotrexate Fluorouracil (CMF) in addition to tamoxifen. The 10 years distant disease-free (DDF) survival based on RS were high RS-88 versus 60% (relative risk [RR] 0.26, 95% confidence interval [CI] 0.13–0.53); low RS-96 versus 97% (RR 1.31, 95% CI 0.46–3.78); and intermediate RS – 90 versus 91% (RR 0.61, 95% CI 0.24–1.59). In SWOG 8814 trial node positive, ER-positive breast cancer were treated with CAF in addition to tamoxifen that resulted in DDF at 10 years high RS – DDF 55 versus 43%, (hazard ratio [HR] 0.59, 95% CI 0.35–1.01) and overall survival-73 versus 54%, (HR 0.56, 95% CI 0.31–1.02). These results were not seen among women with a low or intermediate RS.
Various research groups using supervised approaches have developed multigene signatures designed to predict response in patients receiving either chemotherapy or endocrine therapy. Several studies have attempted to chemotherapy by comparing gene expression profiles between high sensitivity and low-responsive tumors.52,53,54,55,56,57,58 The majority of the studies focused on identification of multigene signatures predicting response to neoadjuvant chemotherapy on the analyzed tumor samples obtained from biopsies taken at diagnosis before initiation of chemotherapy as shown in Table 5.
Research groups |
Gene signature |
Technology platform |
Neo-adjuvant chemotherapy |
Accuracy (%) |
---|---|---|---|---|
Chang et al.52 |
92 genes |
cDNA microarray |
Docetaxel |
88 |
Ayers et al.53 |
74 genes |
cDNA microarray |
T/FAC |
78 |
Iwao-Koizumi et al.54 |
85 genes |
RT-PCR |
Docetaxel |
80.7 |
Hess et al.55 |
30 genes |
cDNA microarray |
T/FAC |
76 |
Thuerigen et al.56 |
512 genes |
cDNA microarray |
G-ET |
88 |
Farmer et al.57 |
Stromal metagenes |
cDNA microarray |
FEC |
65 |
Gianni et al.58 |
86 genes |
RT-PCR/DNA microarray |
TA |
- |
cDNA - Complementary DNA; RT-PCR - Reverse-transcriptase polymerase chain reaction; FAC - Fluorouracil, doxorubicin, cyclophosphamide; FEC - Fluorouracil, epirubicin, and cyclophosphamide; G-ET - Gemcitabine, Epirubicin, Docetaxel; TA - Paclitaxel, Doxorubicin
Despite the promising results, the signatures of chemotherapy sensitivity have so far had limited use in clinical practice. None of the different predictors of chemosensitivity is commercially available, and additional evidence is still required before they can be implemented in clinical practice.
Multigene markers of response to endocrine therapy
ER status has an important negative predictive value for evaluating the response to antiestrogen therapy. However, ER expression alone fails to predict which ER-positive tumor will respond or be resistant to different modalities of endocrine therapies. Various microarray-based gene expression signatures to predict the outcome of tamoxifen-treated patients have been developed by various research groups as shown in Table 6.
Research group |
Signature |
Technology platform |
---|---|---|
Symmans et al.59 |
SET index (165 genes) |
Microarray |
Jansen et al.60 |
44 genes |
Microarray |
Oh et al.61 |
822 genes |
Microarray |
Loi et al.62 |
181 genes |
Microarray |
SET - Sensitivity to endocrine therapy
Sensitivity to endocrine therapy (SET) index was developed in a large series of ER-positive breast cancers was based on the principle that expression of genes correlated with ER may better predict response to endocrine treatment than ER expression alone.59 Analysis of 165 genes coexpressed with ER, the SET index seems to be predictive of benefit from endocrine therapy independently of the inherent prognosis of the tumor and to identify a subset of tumors associated with an excellent prognosis and no relapse. Studies evaluating the clinical relevance of the SET index are warranted to expand its indications in clinical practice.
Future Trends
Microarray-based GEP analysis has undoubtedly influence our understanding of breast cancer biology, the concept of the breast cancer heterogeneity and molecular subtypes. The incorporation of molecular assays into the treatment planning strategy of breast cancer continues to be a work in progress, and this approach is evolving quickly due to strong scientific evidence and expected to be to become the standard of practice in the near future. Newer technologies like next generation sequencing, where repertoire of genetic aberrations in a tumor can be assessed in a single experiment, are expected to complement the microarray based GEP, especially for prognosis and prediction. Integrative approaches, where combining genetic, epigenetics, transcriptomic, proteomic, and metabolomic information, are comprehensive in nature may lead to the creation of a newer breast cancer classification systems with a better understanding of the biology of the disease that have more clinical relevance.
Financial support and sponsorship
Nil.
Conflicts of interest
There are no conflicts of interest.
References
- Phenotypic and molecular characterization of the claudin-low intrinsic subtype of breast cancer. Breast Cancer Res. 2010;12:R68.
- [Google Scholar]
- Expression of luminal and basal cytokeratins in human breast carcinoma. J Pathol. 2004;203:661-71.
- [Google Scholar]
- Classification and risk stratification of invasive breast carcinomas using a real-time quantitative RT-PCR assay. Breast Cancer Res. 2006;8:R23.
- [Google Scholar]
- Gene expression patterns of breast carcinomas distinguish tumor subclasses with clinical implications. Proc Natl Acad Sci U S A. 2001;98:10869-74.
- [Google Scholar]
- Repeated observation of breast tumor subtypes in independent gene expression data sets. Proc Natl Acad Sci U S A. 2003;100:8418-23.
- [Google Scholar]
- Breast cancer classification and prognosis based on gene expression profiles from a population-based study. Proc Natl Acad Sci U S A. 2003;100:10393-8.
- [Google Scholar]
- Conservation of breast cancer molecular subtypes and transcriptional patterns of tumor progression across distinct ethnic populations. Clin Cancer Res. 2004;10:5508-17.
- [Google Scholar]
- An immune response gene expression module identifies a good prognosis subtype in estrogen receptor negative breast cancer. Genome Biol. 2007;8:R157.
- [Google Scholar]
- Supervised risk predictor of breast cancer based on intrinsic subtypes. J Clin Oncol. 2009;27:1160-7.
- [Google Scholar]
- The molecular portraits of breast tumors are conserved across microarray platforms. BMC Genomics. 2006;7:96.
- [Google Scholar]
- A multigene assay to predict recurrence of tamoxifen-treated, node-negative breast cancer. N Engl J Med. 2004;351:2817-26.
- [Google Scholar]
- Association between the 21-gene recurrence score assay and risk of locoregional recurrence in node-negative, estrogen receptor-positive breast cancer: Results from NSABP B-14 and NSABP B-20. J Clin Oncol. 2010;28:1677-83.
- [Google Scholar]
- Prediction of risk of distant recurrence using the 21-gene recurrence score in node-negative and node-positive postmenopausal patients with breast cancer treated with anastrozole or tamoxifen: A TransATAC study. J Clin Oncol. 2010;28:1829-34.
- [Google Scholar]
- Gene expression and benefit of chemotherapy in women with node-negative, estrogen receptor-positive breast cancer. J Clin Oncol. 2006;24:3726-34.
- [Google Scholar]
- Prognostic and predictive value of the 21-gene recurrence score assay in postmenopausal women with node-positive, oestrogen-receptor-positive breast cancer on chemotherapy: A retrospective analysis of a randomised trial. Lancet Oncol. 2010;11:55-65.
- [Google Scholar]
- Gene expression profiles in paraffin-embedded core biopsy tissue predict response to chemotherapy in women with locally advanced breast cancer. J Clin Oncol.. 2005;23:7265-77. et al.
- [Google Scholar]
- Prospective Validation of a 21-Gene Expression Assay in Breast Cancer. N Engl J Med. 2015;373:2005-2014. et al.
- [Google Scholar]
- Prognostic utility of the 21-gene assay in hormone receptor-positive operable breast cancer compared with classical clinicopathologic features. J Clin Oncol. 2008;26:4063-71. Erratum in: J Clin Oncol 2009;27:3566.
- [Google Scholar]
- Gene expression profiling predicts clinical outcome of breast cancer. Nature. 2002;415:530-6.
- [Google Scholar]
- A gene-expression signature as a predictor of survival in breast cancer. N Engl J Med. 2002;347:1999-2009.
- [Google Scholar]
- Validation and clinical utility of a 70-gene prognostic signature for women with node-negative breast cancer. J Natl Cancer Inst. 2006;98:1183-92.
- [Google Scholar]
- The 70-gene prognosis signature predicts early metastasis in breast cancer patients between 55 and 70 years of age. Ann Oncol. 2010;21:717-22.
- [Google Scholar]
- EORTC Trial 10041 (BIG 3-04) – MINDACT (Microarray in Node-Negative and 1 to 3 Positive Lymph Node Disease May Avoid ChemoTherapy) A Prospective Randomized Study Comparing the 70-Gene Signature with the Common Clinical-Pathological Criteria in Selecting Patients for Adjuvant Chemotherapy in Breast Cancer with 0-3 Positive Nodes. Available from: http://www.eortc.be/services/unit/mindact/MINDACT_websiteii.asp [Last accessed on 2009 Sep 10].
- [Google Scholar]
- The 70-gene signature as a response predictor for neoadjuvant chemotherapy in breast cancer. Breast Cancer Res Treat. 2010;119:551-8.
- [Google Scholar]
- The predictive value of the 70-gene signature for adjuvant chemotherapy in early breast cancer. Breast Cancer Res Treat. 2010;120:655-61.
- [Google Scholar]
- Robustness, scalability, and integration of a wound-response gene expression signature in predicting breast cancer survival. Proc Natl Acad Sci U S A. 2005;102:3738-43.
- [Google Scholar]
- The prognostic role of a gene signature from tumorigenic breast-cancer cells. N Engl J Med. 2007;356:217-26.
- [Google Scholar]
- Comparison of PAM50 risk of recurrence score with oncotype DX and IHC4 for predicting risk of distant recurrence after endocrine therapy. J Clin Oncol. 2013;31:2783-90. et al.
- [Google Scholar]
- A comparison of PAM50 intrinsic subtyping with immunohistochemistry and clinical prognostic factors in tamoxifen-treated estrogen receptor-positive breast cancer. Clin Cancer Res. 2010;16:5222-32.
- [Google Scholar]
- A 50-gene intrinsic subtype classifier for prognosis and prediction of benefit from adjuvant tamoxifen. Clin Cancer Res. 2012;18:4465-72.
- [Google Scholar]
- Comparison of PAM50 risk of recurrence score with oncotype DX and IHC4 for predicting risk of distant recurrence after endocrine therapy. J Clin Oncol. 2013;31:2783-90.
- [Google Scholar]
- Identifying clinically relevant prognostic subgroups of postmenopausal women with node-positive hormone receptor-positive early-stage breast cancer treated with endocrine therapy: A combined analysis of ABCSG-8 and ATAC using the PAM50 risk of recurrence score and intrinsic subtype. Ann Oncol. 2015;26:1685-91. et al.
- [Google Scholar]
- Predicting distant recurrence in receptor-positive breast cancer patients with limited clinicopathological risk: Using the PAM50 Risk of Recurrence score in 1478 postmenopausal patients of the ABCSG-8 trial treated with adjuvant endocrine therapy alone. Ann Oncol. 2014;25:339-45.
- [Google Scholar]
- Strong time dependence of the 76-gene prognostic signature for node-negative breast cancer patients in the TRANSBIG multicenter independent validation series. Clin Cancer Res. 2007;13:3207-14.
- [Google Scholar]
- Gene expression profiling in breast cancer: Understanding the molecular basis of histologic grade to improve prognosis. J Natl Cancer Inst. 2006;98:262-72.
- [Google Scholar]
- Comparison of the prognostic value of genomic grade index, Ki67 expression and mitotic activity index in early node-positive breast cancer patients. Ann Oncol. 2013;24:625-32.
- [Google Scholar]
- A five-gene molecular grade index and HOXB13:IL17BR are complementary prognostic factors in early stage breast cancer. Clin Cancer Res. 2008;14:2601-8.
- [Google Scholar]
- HOXB13-to-IL17BR expression ratio is related with tumor aggressiveness and response to tamoxifen of recurrent breast cancer: A retrospective study. J Clin Oncol. 2007;25:662-8.
- [Google Scholar]
- A new molecular predictor of distant recurrence in ER-positive, HER2-negative breast cancer adds independent information to conventional clinical risk factors. Clin Cancer Res. 2011;17:6012-20.
- [Google Scholar]
- The effect of the stromal component of breast tumours on prediction of clinical outcome using gene expression microarray analysis. Breast Cancer Res. 2006;8:R32.
- [Google Scholar]
- Molecular characterization of the tumor microenvironment in breast cancer. Cancer Cell. 2004;6:17-32.
- [Google Scholar]
- Biological processes associated with breast cancer clinical outcome depend on the molecular subtypes. Clin Cancer Res. 2008;14:5158-65.
- [Google Scholar]
- Stromal gene expression predicts clinical outcome in breast cancer. Nat Med. 2008;14:518-27.
- [Google Scholar]
- A stroma-related gene signature predicts resistance to neoadjuvant chemotherapy in breast cancer. Nat Med. 2009;15:68-74.
- [Google Scholar]
- An immune response gene expression module identifies a good prognosis subtype in estrogen receptor negative breast cancer. Genome Biol. 2007;8:R157.
- [Google Scholar]
- T-cell metagene predicts a favorable prognosis in estrogen receptor-negative and HER2-positive breast cancers. Breast Cancer Res. 2009;11:R15.
- [Google Scholar]
- PIK3CA mutations associated with gene signature of low mTORC1 signaling and better outcomes in estrogen receptor-positive breast cancer. Proc Natl Acad Sci U S A. 2010;107:10208-13.
- [Google Scholar]
- Insulin-like growth factor-I activates gene transcription programs strongly associated with poor breast cancer prognosis. J Clin Oncol. 2008;26:4078-85.
- [Google Scholar]
- Oncogenic pathway signatures in human cancers as a guide to targeted therapies. Nature. 2006;439:353-7.
- [Google Scholar]
- Gene expression profiling for the prediction of therapeutic response to docetaxel in patients with breast cancer. Lancet. 2003;362:362-9.
- [Google Scholar]
- Gene expression profiles predict complete pathologic response to neoadjuvant paclitaxel and fluorouracil, doxorubicin, and cyclophosphamide chemotherapy in breast cancer. J Clin Oncol. 2004;22:2284-93.
- [Google Scholar]
- Prediction of docetaxel response in human breast cancer by gene expression profiling. J Clin Oncol. 2005;23:422-31.
- [Google Scholar]
- Pharmacogenomic predictor of sensitivity to preoperative chemotherapy with paclitaxel and fluorouracil, doxorubicin, and cyclophosphamide in breast cancer. J Clin Oncol. 2006;24:4236-44.
- [Google Scholar]
- Gene expression signature predicting pathologic complete response with gemcitabine, epirubicin, and docetaxel in primary breast cancer. J Clin Oncol. 2006;24:1839-45.
- [Google Scholar]
- A stroma-related gene signature predicts resistance to neoadjuvant chemotherapy in breast cancer. Nat Med. 2009;15:68-74.
- [Google Scholar]
- Gene expression profiles in paraffin-embedded core biopsy tissue predict response to chemotherapy in women with locally advanced breast cancer. J Clin Oncol. 2005;23:7265-77.
- [Google Scholar]
- Genomic index of sensitivity to endocrine therapy for breast cancer. J Clin Oncol. 2010;28:4111-9.
- [Google Scholar]
- Molecular classification of tamoxifen-resistant breast carcinomas by gene expression profiling. J Clin Oncol. 2005;23:732-40.
- [Google Scholar]
- Estrogen-regulated genes predict survival in hormone receptor-positive breast cancers. J Clin Oncol. 2006;24:1656-64.
- [Google Scholar]
- Predicting prognosis using molecular profiling in estrogen receptor-positive breast cancer treated with tamoxifen. BMC Genomics. 2008;9:239. et al.
- [Google Scholar]