Artificial Intelligence in Oncology: Potential and Pitfalls
Address for correspondence Munish Gairola, MD, DNB, Department of Radiation Oncology, Rajiv Gandhi Cancer Institute and Research Centre, New Delhi, India. gairola.munish@rgcirc.org
This article was originally published by Thieme Medical and Scientific Publishers Private Ltd. and was migrated to Scientific Scholar after the change of Publisher.
Artificial intelligence (AI) has occupied the consciousness of successive generations of computer scientists, science fiction fans, and medical researchers alike, since the inception of the term in 1956.1 The concept of AI as envisioned in popular culture is that of intelligent machines that can interpret the world as humans do, understand language, and learn from real world examples. While this specific vision of machines being able to replicate human thoughts, emotions, and reasons, remains for now in the realm of science fiction, narrower applications of AI that can perform specific tasks as good as humans are poised to transform medicine at the basic, clinical, management, and financial levels.
Terminology surrounding these technologies continues to evolve and can often be a source of confusion for noncomputer scientists. Fig. 1 provides a broad overview. Data science is the broad field within which the subfields of AI, machine learning (ML), and deep learning (DL) reside, with each successive layer adding more complexity than the former. ML employs algorithms which can learn complex relationships or patterns from empirical data and make accurate decisions without coding specific instructions to accomplish the assigned task.2 The algorithm is “trained” using large volumes of data, which confer it the ability to “learn” how to perform the assigned task, under human supervision. In contrast, DL algorithms can be largely automatic once set in motion, learning intricate patterns from even raw data with minimal human intervention, and improving continuously.3 The difference between the two is highlighted in Fig. 2.
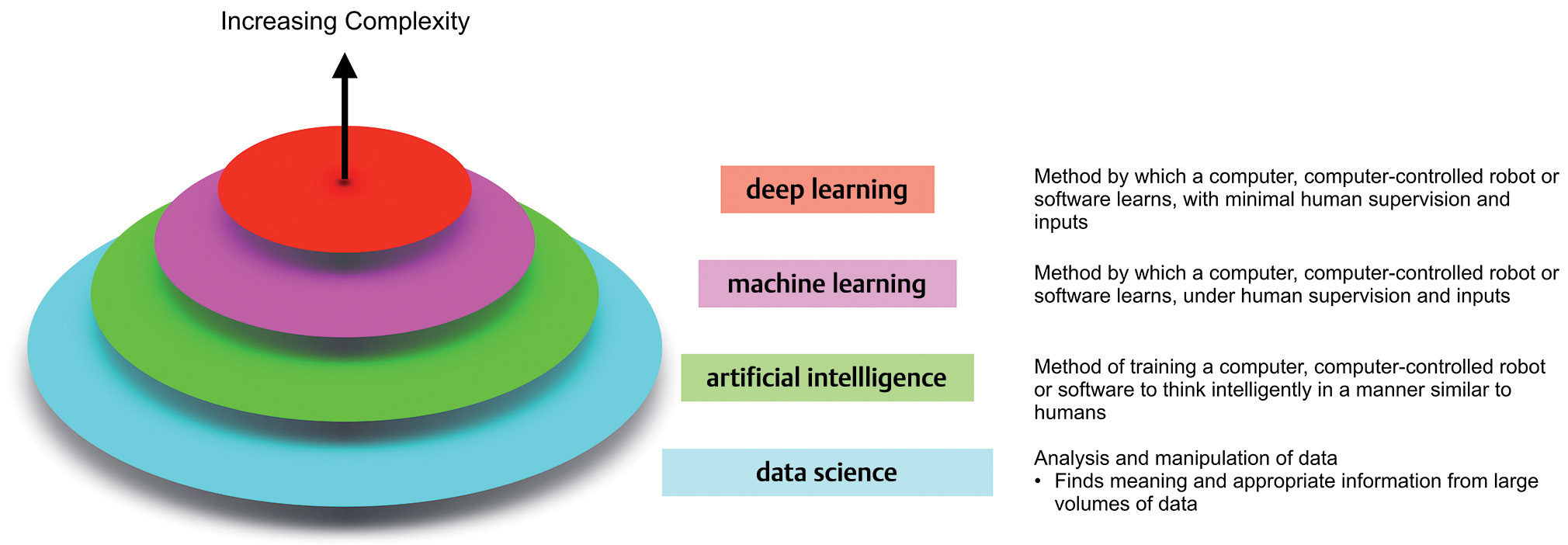
-
Fig. 1 An overview of the field of data science and its organization.
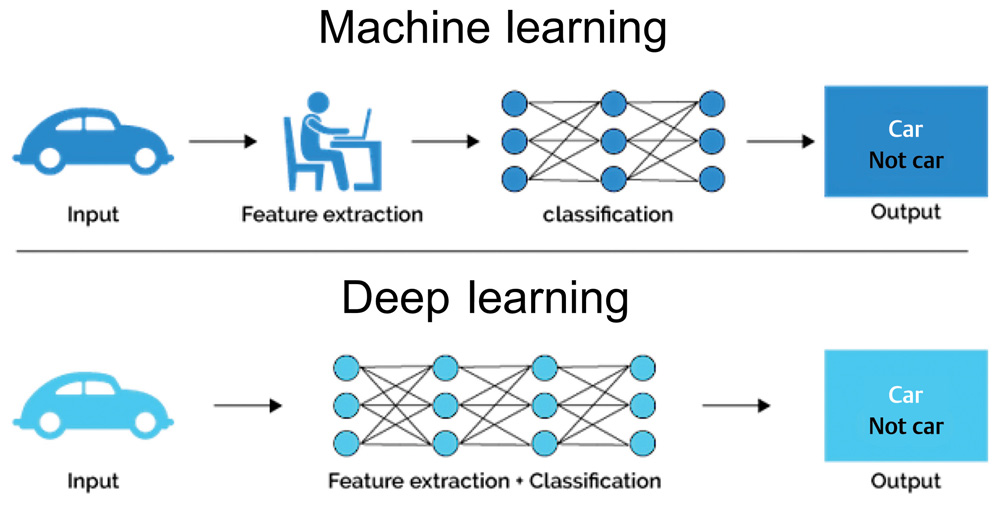
-
Fig. 2 The difference between ML and DL. While ML requires human intervention and supervision, DL functions independent of human intervention. The logic (classification) of both algorithms is impossible to understand. DL, deep learning; ML, machine learning.
The potential and pitfalls of applying AI in oncology is exemplified by IBM’s Watson for Oncology (WFO) program, operated from Memorial Sloan Kettering Cancer Center (MSKCC) in New York and deployed in more than 230 hospitals across the world.4 Initially, envisioned as a cloud based super-computer embedded with artificial intelligence, AI could shift through massive volume of data (from doctor’s notes to medical studies and clinical guidelines) to generate insights and identify, provocatively, new approaches to cancer care. However, till date, the only evidence, citing its capabilities, has focused on concordance analyses and demonstrated that it is competent (at best) in applying existing standard of care, not that it can improve them.5,6 Further shortcomings pertain to the training dataset being composed of synthetic cases rather than real world patients, resulting in treatment recommendations based on the preferences of a small subset of oncologists practicing at MSKCC.6 In the current landscape of data science, it would be classified as a primitive form of ML.
More sophisticated applications of AI are emerging at a rapid pace in all fields of medicine, and oncology is not an exception. These can be broadly classified into identification-, prediction- or discovery-focused applications of AI, and the distinction between them is often not clear. Identification-type applications can detect regions of interest (ROI; e.g., classification of moles on the basis of their malignant potential, malignant cells in histopathology images, or malignant appearing lesions on a radiological imaging modality such as mammogram/computed tomography [CT]/magnetic resonance imaging [MRI]) and classify these ROIs as malignant or benign.7,8 Prediction-focused applications result in identification of features (derived from quantifiable measures of the patient as a whole, the tumor, the clinical care received, or any aspect thereof), which can determine an outcome of interest.9 Finally, discovery-focused applications identify previously unknown patient subgroups based on outcomes, assist in drug design and modeling based on analyzing a multitude of omics datasets (useful in translation oncology).10
While no oncological subspecialty is immune to the effects of wider AI integration in the near future, the earliest to be challenged will likely be diagnostic subspecialties, namely, pathology and radiodiagnosis, followed closely by imaging-based subspecialties, such as radiation oncology (including medical physics and dosimetry). The examples cited here are an introductory representation of the potential applications of AI and a full review of the same is beyond the scope of this article.
It is a foregone conclusion that the impending future of AI integration into oncological practice will be met with either resistance or enthusiasm from practitioners. Gazing into the crystal ball, one can envision an optimized health care delivery system in which patient care plans would be generated by algorithmically driven software and subsequently refined by oncologists based on their patient outcomes to maximize clinical benefit. Delivery of care would then be managed by a tiered team of clinicians based on their expertise. This human–machine symbiosis has been termed the centaur model.11
A key barrier to adoption will be the “black-box” effect, which is the inability of AI algorithms to explain their predictions.12 In all fairness, oncologists are also unable to perfectly explain their own judgments, which may be based on experience/intuition and the associated cognitive biases, but as new technology, the burden of proof is on AI to account for its predictions. Taking this argument one step further, if oncologists do not understand why the algorithm recommended a particular line of management, then why should patients trust the recommendation? Taken to the extreme, who is ultimately responsible for an algorithmic error in diagnosis or treatment? The doctor or the data scientist?
The application of AI in oncology holds tremendous potential and, although it still remains to be explored fully, the benefits cannot be ignored. However, as clinicians we also have every right to be skeptical of inscrutable algorithms, and if they are demonstrably outperforming our best judgment, it is our duty to ask why.
Conflict of Interest
None declared.
References
- The dartmouth college artificial intelligence conference: the next fifty years. AI Mag. 2006;27(05):87-91.
- [Google Scholar]
- Envisioning Watson as a rapid-learning system for oncology. J Oncol Pract. 2013;9(03):155-157.
- [Google Scholar]
- Watson for oncology and breast cancer treatment recommendations: agreement with an expert multidisciplinary tumor board. Ann Oncol. 2018;29(02):418-423.
- [Google Scholar]
- Anderson breaks with IBM Watson, raising questions about artificial intelligence in oncology. J Natl Canc Inst 2017;109(5)
- Dermatologist-level classification of skin cancer with deep neural networks. Nature 2017:115-118. 542(7639):
- [Google Scholar]
- 3D multi-view convolutional neural networks for lung nodule classification. PLoS One. 2017;12(11):e0188290.
- [Google Scholar]
- Machine learning approaches for predicting radiation therapy outcomes: a clinician’s perspective. Int J Radiat Oncol Biol Phys. 2015;93(05):1127-1135.
- [Google Scholar]
- Computational protein design with deep learning neural networks. Sci Rep. 2018;8(01):6349.
- [Google Scholar]
- Human-machine collaboration in cancer and beyond: the centaur care model. JAMA Oncol. 2017;3(10):1303-1304.
- [Google Scholar]
- Clinical applications of machine learning algorithms: beyond the black box. BMJ. 2019;364:l886.
- [Google Scholar]